On women's health & Responsible AI
- ellencoughlan
- Mar 5
- 5 min read
Updated: Mar 7
Lizzie Remfry, Statistical Methods theme team
Ellen Coughlan, Social Justice theme team
On a sunny day in London recently, a few DSxHE community members and the great and good of data science and healthcare assembled at the Royal College of Obstetricians and Gynaecologists for the Responsible AI: Women and Healthcare Conference. Hosted by the Department of Health and Social Care, it was an insightful day that brought together government, NHS, policy makers, academics and the public, with a keynote from the one and only Caroline Criado-Perez. This conference provided a powerful space to discuss how AI can play a role in transforming healthcare for women, and how to do this responsibly! Ahead of International Women's Day on 8th March 2025, we're reflecting on what we learned and what kind of change we think is needed.
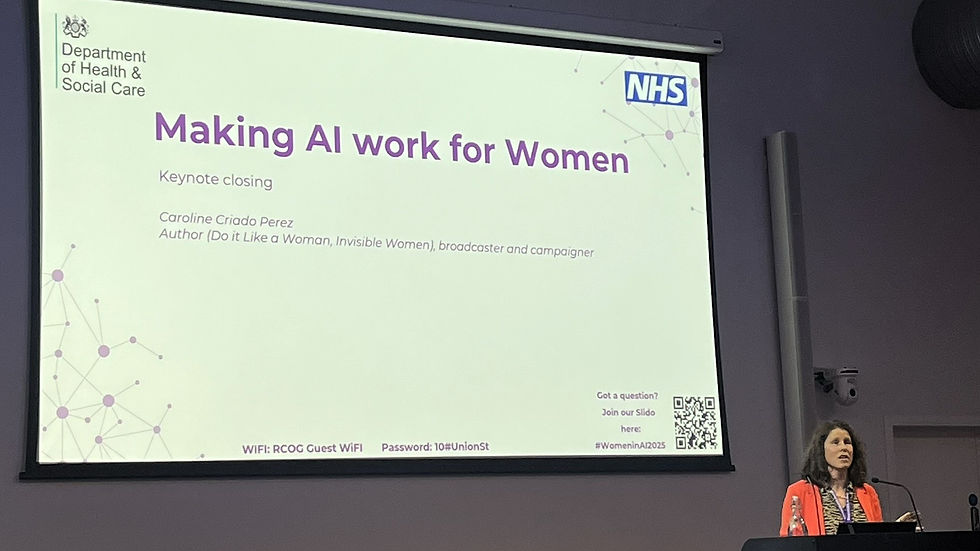
Why is this important?
Women’s health conditions are chronically underfunded, with only 1% of global health funding spent on female specific conditions, and diseases that affect mainly women are underfunded compared to their burden they place on the population as a whole, even though women make up 51% of the world's population. Not only do we not fund research into women’s health conditions, when research is conducted, such as clinical trials to develop new drugs, the majority of participants are male, even in research areas that predominantly affect women. This means that often medication doesn’t work as well in women, women may experience significantly more side effects or women receive incorrect dosages.
As AI becomes widely used in health research and healthcare delivery there are major concerns that it will worsen existing health inequalities. As AI models are typically trained on historical data, the inequalities we see around women’s health may only get worse.
This conference was a space to discuss many of these thorny issues, and some DSxHE members reflected on their day:
What’s one new thing that you learned from the speakers?
Emma Karoune, Turing: “That the UK could save £36bn per year in the NHS by closing the women’s health gap - not a small amount!”
Lizzie Remfry, DSxHE organiser: "Women and men feel quite differently about the use of AI in their health, a survey from the Health Foundation found that women are less likely to think AI will improve care quality (28%) compared to men (39%). It would be interesting to dig into this finding to explore why men and women hold differing views about the utility of AI in healthcare."
Are you taking away any actions from the conference?
Nell Thornton, Health Foundation: “A quote from the day that stood out to me was “I’m not going to be able to solve problems for someone who has lived a very different life”. Working in research and policy influencing positions often means we’re advocating for people who are not in those spaces, and we bring our own assumptions and lived experiences to how we see the world. An action I took from the day was to step back, reconnect with my own reflexive practice, and recommit to ensuring I am amplifying voices rather than speaking for them.”
Emma Karoune, Turing: “Many of the speakers talked about the need to collect more data such as involving more women in clinical trials, but there was also the mention of reusing data and the potential of using AI to debias data or fix missingness in data - these are aspects of research that we have addressed at Turing during Covid research and as part of the Turing-Roche partnership, so I’m thinking about what else could be done to help close this gap in health provision. If you're interested in our work, you can find our Clinical AI interest group here.”
What topic do you hope is discussed further at the next conference?
Maxine Mackintosh, Sciana Fellow: “A central theme was the precision of sex and gender, and one of the key takeaways was Caroline Criado-Perez’s call to “sex-disaggregate your data.” Ideally, disaggregating data by gender should be a deliberate choice: it matters when one intends to capture the lived experience of gender, ie how someone experiences being a woman or is perceived as one, just as there are inherent biological aspects related to being female. However, the practice is often applied indiscriminately, serving as a proxy for other factors such as body size or family structure, which risks embedding sexist assumptions into our models, especially when gender or sex may not be the primary factor influencing an outcome. A more thoughtful approach would be to advocate for disaggregation only when sex or gender is the true and central focus of the inquiry. This not only avoids the inadvertent encoding of biases but also ensures that data analyses remain true to their intended purpose. In this spirit, a provocative yet essential call to action could be precision in your data disaggregation practices. Do it only when you are truly investigating aspects where sex or gender is fundamentally relevant.”
Nell Thornton, Health Foundation: “It was great to hear speakers discuss how historic biases are being recreated and intensified in the data that are powering AI systems, and some of the things we need to think about to reduce bias and its resulting discrimination. But how do we use AI to reduce inequalities, and not just mitigate them? How do we move towards uses of AI that are truly responsible, and working for all people, society and the planet?”
If you could have had a dream speaker on the panel who would it have been?
Maxine Mackintosh, Sciana Fellow: “The question of sex-disaggregation of data is in line with the work of people like Angela Saini (a DSxHE favourite!) who regularly reminds us of the importance of careful, context-specific analysis. Crude disaggregation by complex social (or even biological) factors, may artificially create the appearance of a sex or gender difference where there is none, and in turn, convert a well-meaning analysis, into a sexist analysis. Get her on stage next time!”
Nell Thornton, Health Foundation: “There are some incredible women working in this space, and working to challenge the status quo in what is a very imbalanced sector. There are too many to name here, but I would love to hear from Joy Buolamwini, who was fundamental in highlighting how machine learning algorithms can discriminate based on classes like race and gender, and Timnit Gebru who launched the Distributed Artificial Intelligence Research Institute (DAIR), which documents the effect of AI on marginalised groups.”
Comments